Equipo de investigación
Equipo de Trabajo
Asesor Científico
El principal objetivo de este proyecto diseñar una infraestructura IoT de altas prestaciones, diseñada para analizar ingentes cantidades de datos mediante técnicas de aprendizaje máquina en tiempo real. Este objetivo general se divide en los siguientes objetivos específicos:
- Análisis, diseño y evaluación de infraestructuras IoT en entornos reales de aplicación. En este objetivo se pretende desplegar una infraestructura IoT en entornos reales que permita la medición de diferentes variables contextuales y ambientales, como por ejemplo temperatura ambiente, velocidad del viento, humedad, sonido ambiental, etc). Esta información se deberá enviar, en primera instancia, a un servidor donde se almacenará para su posterior procesamiento. Además, se evaluará diferentes alternativas hardware para realizar el despliegue y que garanticen la optimización en rendimiento y consumo energético.
- Diseño de estrategias de runtime para habilitar computación en el edge. En este objetivo se pretende habilitar la computación en el extremo de la infraestructura IoT para facilitar una computación multiescala donde se reduzca el ancho de banda necesario en las comunicaciones, así como dar respuesta en tiempo real en ciertos escenarios.
- Diseño de algoritmos de machine learning mediante técnicas de codiseño hardware-software. Se diseñarán técnicas de machine learning multiescala eficiente adaptadas a la nueva infraestructura distribuida diseñada. Dichas técnicas permitirán la detección de eventos y situaciones de interés con baja latencia relativos al entorno real de aplicación.
The main objective of this project is to design a high performance IoT infrastructure, designed to analyse huge amounts of data using real-time machine learning techniques. This general objective is divided into the following specific objectives:
- Analysis, design and evaluation of IoT infrastructures in real application environments. This objective is to deploy an IoT infrastructure in real environments that allows the measurement of different contextual and environmental variables, such as ambient temperature, wind speed, humidity, ambient sound, etc.) This information must be sent, in the first instance, to a server where it will be stored for later processing. In addition, different hardware alternatives will be evaluated to carry out the deployment and to guarantee the optimization in performance and energy consumption.
- Design of runtime strategies to enable edge computing. This objective aims to enable computing at the edge of the IoT infrastructure to facilitate multiscale computing where the bandwidth needed is reduced by communications, as well as providing real-time response in certain scenarios.
- Design of machine learning algorithms using hardware-software coding techniques. Efficient multiscale machine learning techniques will be designed, adapted to the new distributed infrastructure designed. These techniques will allow the detection of events and situations of interest with low latency related to the real application environment.
2021
- Andreo-Martínez, P., Ortiz-Martínez, V. M., Muñoz, A., Menchón-Sánchez, P., & Quesada-Medina, J. (2021). A web application to estimate the carbon footprint of constructed wetlands. Environmental Modelling & Software, 135, 104898.
- Sidhu, N., Pons-Buttazzo, A., Muñoz, A., & Terroso-Saenz, F. (2021). A Collaborative Application for Assisting the Management of Household Plastic Waste through Smart Bins: A Case of Study in the Philippines. Sensors, 21(13), 4534.
- Cecilia, J. M., Cano, J., Calafate, C. T., Manzoni, P., Perinan-Pascual, C., Arcas-Tunez, F., & Munoz-Ortega, A. (2021). WATERSensing: A smart warning system for natural disasters in Spain. IEEE Consumer Electronics Magazine, 10(6), 89 - 96.
- Terroso-Sáenz, F., Muñoz, A., Fernández-Pedauye, J., & Cecilia, J. M. (2021). Human Mobility Prediction With Region-Based Flows and Water Consumption. IEEE Access, 9, 88651-88663.
- Guillén, M. A., Llanes, A., Imbernón, B., Martínez-España, R., Bueno-Crespo, A., Cano, J. C., & Cecilia, J. M. (2021). Performance evaluation of edge-computing platforms for the prediction of low temperatures in agriculture using deep learning. The Journal of Supercomputing, 77(1), 818-840.
- Orenes-Vera, M., Terroso-Saenz, F., and Valdes-Vela, M. (2021). RECITE: A Framework for User Trajectory Analysis in Cultural Sites. Journal of Ambient Intelligence and Smart Environments, 13(5), 389-409.
- Arcas-Tunez, F., & Terroso-Saenz, F. (2021). Forest path condition monitoring based on crowd-based trajectory data analysis. Journal of Ambient Intelligence and Smart Environments, 13(1), 1-18.
- García-Cremades, S., Morales-García, J., Hernández-Sanjaime, R., Martínez-España, R., Bueno-Crespo, A., Hernández-Orallo, E., ... & Cecilia, J. M. (2021). Improving prediction of COVID-19 evolution by fusing epidemiological and mobility data. Scientific Reports, 11(1), 1-16.
- Nakamura, K., Hernández, D., Cecilia, J. M., Manzoni, P., Zennaro, M., Cano, J. C., & Calafate, C. T. (2021). LADEA: A Software Infrastructure for Audio Delivery and Analytics. Mobile Networks and Applications, 1-7.
- Cebrian, J. M., Imbernón, B., Soto, J., & Cecilia, J. M. (2021). Evaluation of Clustering Algorithms on HPC Platforms. Mathematics, 9(17), 2156.
- Terroso-Saenz, F., Albaladejo, A., Llanes, A., Sidhu, N., Muñoz, A. (2021). Towards a Monitoring Framework for Users of Retirement Houses with Mobile Sensing. In EAI GOODTECHS 2021, 7th EAI International Conference on Smart Objects and Technologies for Social Good, Aveiro, Portugal, September 2021 (in press)
2020
Cebrian, J. M., Imbernón, B., Soto, J., García, J. M., & Cecilia, J. M. (2020). High-throughput fuzzy clustering on heterogeneous architectures. Future Generation Computer Systems.
Cecilia, J. M. (2020). Guest editors’ note: Special issue on novel high-performance computing algorithms and platforms in bioinformatics. The International Journal of High Performance Computing Applications, 34(1), 3–4
Guillén-Navarro, M. A., Martínez-España, R., Llanes, A., Bueno-Crespo, A., & Cecilia, J. M. (2020). A deep learning model to predict lower temperatures in agriculture. Journal of Ambient Intelligence and Smart Environments, (Preprint), 1-14.
Jimeno-Sáez, P.; Senent-Aparicio, J.; Cecilia, J.M.; Pérez-Sánchez, J. Using Machine-Learning Algorithms for Eutrophication Modeling: Case Study of Mar Menor Lagoon (Spain). Int. J. Environ. Res. Public Health 2020, 17, 1189.
Navarro, J. M., Martínez-España, R., Bueno-Crespo, A., Martínez, R., & Cecilia, J. M. (2020). Sound Levels Forecasting in an Acoustic Sensor Network Using a Deep Neural Network. Sensors, 20(3), 903.
Terroso-Saenz, F., & Munoz, A. (2020). Land use discovery based on Volunteer Geographic Information classification. Expert Systems with Applications, 140, 112892.
Guillén, M.A., Llanes, A., Imbernón, B., Martínez-España, R., Bueno-Crespo, A., Cano JC., Cecilia, JM. (2020). Performance evaluation of edge-computing platforms for the prediction of low temperatures in agriculture using deep learning. Journal of Supercomputing (in press)
Imbernón, B., Llanes, A., Cutillas-Lozano, J. M., & Giménez, D. (2020). HYPERDOCK: Improving virtual screening through parallel hyperheuristics. The International Journal of High Performance Computing Applications, 34(1), 30-41.
Hernández, M., Cebrián, J. M., Cecilia, J. M., & García, J. M. (2020). Offloading strategies for Stencil kernels on the KNC Xeon Phi architecture: Accuracy versus performance. The International Journal of High Performance Computing Applications, 34(2), 199-207.
Imbernón, B., Serrano, A., Bueno-Crespo, A., Abellán, J. L., Pérez-Sánchez, H., & Cecilia, J. M. (2020). METADOCK 2: a high-throughput parallel metaheuristic scheme for molecular docking. Bioinformatics. (In press)
Cuenca-Jara, J., Terroso-Sáenz, F., Valdés-Vela, M., & Skarmeta, A. F. (2020). Classification of spatio-temporal trajectories from Volunteer Geographic Information through fuzzy rules. Applied Soft Computing, 86, 105916.
Cecilia, J. M., Cano, J. C., Morales-García, J., Llanes, A., & Imbernón, B. (2020). Evaluation of clustering algorithms on GPU-based edge computing platforms. Sensors, 20(21), 6335.
García, J. M., Llanes, A., Tudela, B. I., & Cecilia, J. M. (2020). Performance Evaluation of Clustering Algorithms on GPUs. In Intelligent Environments 2020 Workshop Proceedings of the 16th International Conference on Intelligent Environments (pp. 400-409). IOS Press
Montoya-Belmonte, J., & Navarro, J. M. (2020). Long-Term Temporal Analysis of Psychoacoustic Parameters of the Acoustic Environment in a University Campus Using a Wireless Acoustic Sensor Network. Sustainability, 12(18), 7406
Cecilia, J. M., Cano, J. C., Hernández-Orallo, E., Calafate, C. T., & Manzoni, P. (2020). Mobile crowdsensing approaches to address the COVID'19 pandemic in Spain. IET Smart Cities, 2(2), 58-63.
2019
Cecilia, J. M., & García, J. M. (2019).Re-engineering the ant colony optimization for CMP architectures. The Journal of Supercomputing, 1-22
Contreras, A. V., Llanes, A., Herrera, F. J., Navarro, S., López-Espín, J. J., & Cecilia, J. M. (2019).Enhancing the context-aware FOREX market simulation using a parallel elastic network model. The Journal of Supercomputing, 1-17
Fernández-Soriano, F. L., López, B., Martínez-España, R., Muñoz, A., & Cantabella, M. (2019). Use of Computing Devices as Sensors to Measure Their Impact on Primary and Secondary Students’ Performance. Sensors, 19(14), 3226.
Gams, M., Gu, I. Y. H., Härmä, A., Muñoz, A., & Tam, V. (2019). Artificial intelligence and ambient intelligence. Journal of Ambient Intelligence and Smart Environments, 11(1), 71-86
Guillén‐Navarro, M. A., Martínez‐España, R., López, B., & Cecilia, J. M. (2019). A high‐performance IoT solution to reduce frost damages in stone fruits. Concurrency and Computation: Practice and Experience, e5299.
GUILLEN-NAVARRO, M. A., MARTINEZ-ESPANA, R., aBelen AYUSO aJose, A. B. C., & MORENO, L. (2019, August). An LSTM Deep Learning Scheme for Prediction of Low Temperatures in Agriculture. In Intelligent Environments 2019: Workshop Proceedings of the 15th International Conference on Intelligent Environments (Vol. 26, p. 130). IOS Press.
Intelligence, A., Muñoz, A., & Park, J. Ebook: Agriculture and Environment Perspectives in Intelligent Systems.
Martínez-España, R., Bueno-Crespo, A., Soto, J., Janik, L. J., & Soriano-Disla, J. M. (2019). Developing an intelligent system for the prediction of soil properties with a portable mid-infrared instrument. Biosystems engineering, 177, 101-108
Saadi, H., Taboudjemat, N. N., Rahmoun, A., Pérez-Sánchez, H., & Cecilia, J. M. (2019). Efficient GPU-based parallelization of solvation calculation for the blind docking problem. The Journal of Supercomputing, 1-19
Terroso-Saenz, F., Muñoz, A., & Cecilia, J. M. (2019). QUADRIVEN: A framework for qualitative taxi demand prediction based on time-variant online social network data analysis. Sensors, 19(22), 4882.
A continuación se listan unas métricas que se utilizarán para cuantificar los resultados producidos por este proyecto, junto con los valores que consideramos aceptables para la satisfacción del mismo.
- Desarrollo de los entregables del proyecto (DE) y cumplimiento de hitos (HI)
- Número de publicaciones asociadas con el proyecto (NP)
- Número de asistencias a congresos nacionales y/o internacionales (AC) congresos/año.
- Organización de actividades científicas (OA)
- Número de tesis leídas derivadas de la investigación del proyecto (NT)
- Nuevas colaboraciones internacionales (CI)
- Nuevas colaboraciones nacionales (CN)
- Difusión en prensa de las actividades investigadores del proyecto (DP)
"El mercado de divisas (FOREX) como un modelo elástico de red" - Antonio Vicente Contreras
Directores:
- Jose María Cecilia Canales
- Horacio Emilio Pérez Sánchez
Fecha defensa:
- 12 Abril 2019
Financiado por
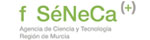